Demand forecasting is a common use case of AI-ML. It can be used to identify areas of improvement and best practices that can help businesses improve its forecasting accuracy. It helps in production planning and determine incremental impacts of new initiatives, plan resources in response to expected demand, and project future budgets.
The following image depicts a simple forecasting cycle. It is all about using historical data, train forecasting model on it, generate forecast to make informed decisions. It’s a cyclic process — when new data arrives, we feed it back into the forecasting model and retrain it so that the model remains up to date.

Demand forecasting cycle
A frequent question we get from customers, consultants and other business experts is “What would you consider a good level of forecast accuracy?”
However, it may not be the most relevant question to raise, though. First of all, forecasting is seldom the objective in any retail or supply chain planning scenario. It is always a means to an end. A forecast is only useful if it helps us accomplish other objectives, like increased shelf availability, accurate production planning, less wastage.
While forecasting is a crucial component of all planning activities, it is only one cog in the machine, which means that other factors like changes in consumer behaviour, economic turndowns, historical data quality can have a significant impact on the forecasting accuracy.
What degree of forecast accuracy can actually be obtained depends on a number of factors. This is one of the reasons why comparing forecast accuracy between businesses, or even between products within the same business, is so challenging.
When integrating demand forecasting systems, it’s essential to understand that they are vulnerable to unpredictable situations. So, the demand forecasting machine learning models should be re-trained according to current reality.
With large forecast horizon, the probability that future demand will be impacted by developments that we are not currently aware of grows noticeably. Weather-dependent demand is a straightforward illustration. If we need to make decisions on what quantities of summer clothes to buy or produce half a year even longer in advance, there is currently no way of knowing what the weather in the summer is going to be.
On the other hand, if we were in charge of overseeing the replenishment of ice cream in grocery shops, we could utilize short-term weather forecast to estimate how much ice cream to transport to each location.
Important features to consider while training ML model for forecasting:
Seasonality
Seasonality refers to the regular and predictable pattern of demand for goods or services that occurs at certain times of the year, such as holidays, weather changes, or cultural events. What’s more, understanding of seasonality for each of your products can give you a head start in demand planning. With a good grasp of how much consumer demand varies, you can select the right demand forecasting models, focus on making plan adjustments and spot true outliers that require further attention.
For example, a business that sells winter clothing may experience a significant increase in demand during the winter months and a decrease in demand during the summer months. Similarly, a business that sells ice cream may experience higher demand during the summer months and lower demand during the winter months.
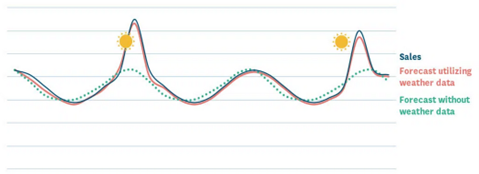
Weather data feature effect
Demand will typically vary from region to region, depending on local calendars and weather. You may need to segment your data geographically to identify seasonality patterns. At the same time, aggregating forecasts to a less granular level — product category instead of product, for example — may make it easier to distinguish seasonal patterns from random noise.
Rather than including only historical time series and demand, incorporate weather data into a machine learning model for demand forecasting as the model can account for the impact of weather on consumer behaviour and give better predictions accordingly. This can lead to help businesses make better decisions about inventory management, marketing strategies, and other aspects of their operations.
Furthermore, weather data can be used to create more granular and accurate models by breaking down the weather into different variables such as temperature, precipitation, humidity, etc. This allows for a more nuanced analysis of how weather affects demand for different products or services.
The following code snippet reads weather data from a csv file and pre-processes it by resampling to daily frequency and imputes the missing values by linear interpolation imputation technique, so that it can used with the historical time-series data to train a forecasting ML model.
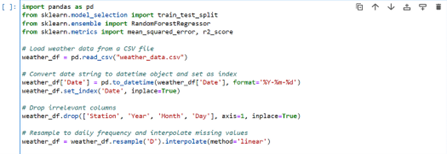
Code snippet to pre-process weather feature
Location
For certain use cases, location is an important feature when training a demand forecasting ML model.
Competition & Vendors: The location of a business and its associated vendors location where a particular product is being sold can play a vital role. Also, if there are many similar businesses in the area, the demand for a particular product or service may be lower than in an area with few or no competitors.
Demographics: The demographics of an area may play a crucial role in determining the demand for a product or service. Different age groups, income levels, and cultural backgrounds have different preferences, and this can be reflected in demand patterns.
Infrastructure: The availability and quality of infrastructure such as roads, transportation, and logistics can also affect demand. Areas with poor infrastructure may have lower demand due to difficulties in accessing or transporting products.
Holidays
Sales patterns can vary significantly during holiday seasons due to changes in consumer behaviour, such as increased shopping activities, changes in preferences, and higher demands for specific products or services.
Including holiday data into the machine learning model helps to improve the accuracy of sales forecasts during these periods, which is essential for businesses to plan their operations and inventory management. Additionally, taking holidays into account in the model can also reveal important insights into customer behaviour and preferences, which can help businesses tailor their marketing strategies to better engage with their target audience.
Trends
One of the benefits of including trends in demand forecasting algorithms is the ability to anticipate market changes and respond accordingly. For example, if a trend analysis indicates a shift in consumer preference for a particular product, the company can adjust their production and marketing strategies to meet the new demand. Additionally, trend features can help identify whether changes in demand are due to short-term fluctuations or long-term changes in the market.
Moreover, trends can also help identify potential bottlenecks in the supply chain, which can help companies optimize their inventory levels and reduce waste. By accurately forecasting future demand, companies can avoid stock outs or overstocking, which can lead to significant losses.
Let’s say you have a dataset that contains daily temperature readings for a specific location over a period of four years. You want to include a feature that represents the trend in temperature over time. One way to do this is to calculate a moving average of the temperature readings for each day, using a window size of, say, 30 days. This will give you a new feature that represents the average temperature over the past 30 days, which can be a good indicator of whether the temperature is trending up or down.
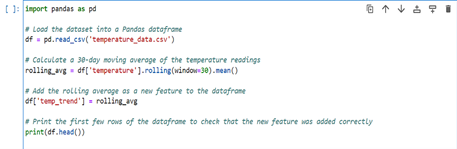
Code snippet to pre-process Temperature trend feature
Above code snippet, reads the ‘temperature_data.csv’ file which contains a column called ‘temperature’ that has the daily temperature readings. The rolling () function is used to calculate a rolling average of the temperature column with a window size of 30. The resulting rolling average is then added as a new column to the data frame, with the name ‘temp_trend’.
Machine learning models/tools that can be used for demand forecasting
• Amazon Forecast: Amazon Forecast is a time-series forecasting service based on machine learning (ML) and built for business metrics analysis. Amazon Forecast uses machine learning (ML) to generate forecasts with just a few clicks, without requiring any prior ML experience. If you have a time-series forecasting use case and you don’t have much experience in machine learning, Amazon Forecast is likely a good choice.
• Amazon SageMaker:It is a fully managed machine learning service. With SageMaker, data scientists and developers can quickly and easily train and test machine learning models, and then directly deploy them into a production-ready hosted environment. If you require more flexibility and control over your machine learning models, add and create features, built relationship between the features then Amazon SageMaker can be a better fit.
• Amazon Deep AR: The Amazon SageMaker Deep AR forecasting algorithm is a supervised learning algorithm for forecasting scalar (one-dimensional) time series using recurrent neural networks (RNN).
• ARIMA: An autoregressive integrated moving average model is a form of regression analysis that gauges the strength of one dependent variable relative to other changing variables. The model’s goal is to predict future data points by examining the differences between values in the series instead of through actual values.
• SARIMA: Seasonal Autoregressive Integrated Moving Average, SARIMA or Seasonal ARIMA, is an extension of ARIMA that explicitly supports univariate time series data with a seasonal component. It adds three new hyperparameters to specify the autoregression (AR), differencing (I) and moving average (MA) for the seasonal component of the series.
• LSTM’s: Long Short — Term Memory is a type of neural network architecture that is commonly used for time series forecasting. LSTM networks are particularly useful for dealing with time series data that have long-term dependencies or complex patterns. The basic idea behind LSTM is to use a series of memory cells to remember and store important information about the input sequence, and then use this information to make predictions about future values in the sequence.
Conclusion
Demand forecasting is a crucial aspect for any business operation, and the use of machine learning algorithms can significantly improve the accuracy and efficiency of the forecasting process. By considering historical data, current trends, and other relevant factors, businesses can better understand their customers’ needs and optimize their production and inventory levels to meet demand while minimizing the loss.
You should ensure that the process of re-training your ML model with new data happens at continuous intervals. The goal of model retraining is to adapt the model to changing patterns in the data, or to improve its accuracy as new data becomes available.
Retraining a model is important because machine learning models are only as good as the data they are trained on. It can also help to address issues such as model drift, where the performance of a model deteriorates over time due to changes in the data distribution.
In conclusion, demand forecasting using machine learning is not a one-time process, but a continuous effort that requires ongoing monitoring and retraining of models on latest available data. By leveraging the latest technologies and insights, companies can stay ahead of the curve and thrive in today’s dynamic and rapidly evolving marketplace.
- Author Yasir Ul Hadi
References:
- https://towardsdatascience.com/sales-forecasting-from-time-series-to-deep-learning-5d115514bfac
- https://www.relexsolutions.com/resources/machine-learning-in-retail-demand-forecasting/
- https://www.relexsolutions.com/resources/measuring-forecast-accuracy/
- https://alloy.ai/choosing-the-right-demand-forecasting-model/
- https://mobidev.biz/blog/machine-learning-methods-demand-forecasting-retail