
Businesses are embracing app modernization on a vast scale. The reason can be to meet greenfield necessities, make business future-ready, or to upgrade monolithic legacy applications. On their journey to modernization, businesses are using containers and Kubernetes as primary technologies to modernize the design and distribution of their applications. The key business goal remains the same, which is to have an all-time-available work system in place. A system that is scalable, portable, flexible, and reliable. Architecture based on microservices and Kubernetes, and the 12 factor app methodology can help achieve such a system.
The 12-factor app style of development surfaced about 10 years ago, much before containers. And, since then the 12 principles of the 12 factor app have become a universal standard for cloud-native app development. The 12 factor app development stages offer a set of guidelines for a proper outline for developing modern microservices. And, Kubernetes is known for being an orchestration platform for containers used to deploy and control these microservices.
The 12-factor app principles:
- Has only one aim: to offer a course of action for cloud-native application development and deployment. They ensure that happens by making applications highly scalable and disposable.
- Help you and your team to embrace DevOps and microservices in the app development process.
- Simplify the process, which increases the development time and reduces the time to market.
- Were designed to build Software as a Service (SaaS) applications by alleviating the difficulties associated with long-term software development.
This article explains how organizations are leveraging the 12-factor app development method and Kubernetes to architect cloud-native apps. Understand how 12 factor app is helping businesses to modernize by establishing scalability, resiliency, robustness, mobility, and reliability across their applications. Let’s get started.
Leveraging 12 Factor App Principles and Kubernetes
1. A single Codebase for Applications, Multiple Deployments

A 12-factor app methodology states that only one Codebase or a set of code repositories should exist. These are deployable multiple times but never have many codebases. If there are any shared codes, they should be factored into libraries and called through the dependency manager.
Multiple deployments of a codebase are possible by making it active across all instances. The only difference is the versions, which are also tracked in the version manager.
Once the code base is in place with the 12-factor app approach, it can be built, released, or run in separate phases in the Kubernetes environment. Kubernetes and containers have text-based representations. The predictable system states are managed by automation tools in separate files. It is better to manage such evolving artifacts with source control. Using a version control system such as Git can help eliminate the introduction of sudden changes and facilitate tracking the changes added to your system.
2. Declare and Isolate Application Dependencies
The 12-factor app methodology uses the declaration and isolation method for application dependencies. Declare any dependencies explicitly and also check them in the version manager. This approach makes it easier to get started and enhances repeatability. It also becomes easy to track any changes made to the dependencies.
Another approach is to package the app and all its dependencies into a container. This makes it possible to remove the app and all its dependencies from its environment. In addition, it ensures that the app functions as expected regardless of the differences in development and staging environments.
3. Archive Config as Environment Variable
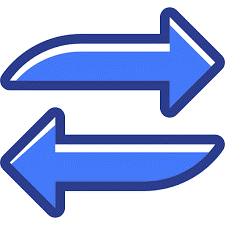
As per 12 factor app principle configs should be archived as environment variables (env vars) but not constants. Env vars are easy to change as the need arises for new code deployments without changing the codes. This flexibility quickens the native-app development process.
Additionally, you can manage env vars independently every time you deploy them. It also becomes easy to scale up as the development process progresses towards completion and deployment.
The 12-factor app strictly separates the application configuration from the code. Kubernetes ConfigMap supports storing configuration by declaring it. This can be helpful for production and development environments that need different configurations to deploy the same code.
4. Backing Services: As Attached Resources, Easy to Swap
Backing services include support applications and systems that your application needs to connect and communicate with, such as databases. They are usually grouped as attached resources that should be accessible when needed.
Modern applications that are microservices-based use backing services. These backing services are handled as attached resources in the 12-factor app. Due to this, in case of any failure, you can simply change the attached resources and not the whole application codebase.
Backing services in the 12-factor app methodology are configurable and easy to change. You can change them from one state to the next as the need arises. The switching is possible by just slightly changing the configuration.
It is the best practice to separate the backing services (such as logging, messaging, databases, third-party services, caching, and others) from the system. And then interact with them through an API. Sticking the APIs to consistent contracts will let you change the basic implementations without highlighting them to clients. Kubernetes ConfigMaps can be used to store connection information to skip building the container image again, in case of any revision in the connection information.
5. Split Build, Release and Run Phases

The 12-factor app methodology distinguishes all the stages of cloud-native app development.
- They changed the codebase to deploy it. And once the first stage is completed and the next starts, you cannot alter the code in the previous one.
- You should build deployable components independent of the environment in the first stage. The second stage involves taking the reusable components already developed and combining them with a specific configuration to match the target environment.
- The last phase is the run stage. It involves packing the entity created in the previous one in a container and running it in the target environment.
Organizations prefer to automate the development and testing tasks with CI/CD toolchains. Splitting your CI/CD pipeline into a series of sequential tasks can increase productivity. It helps to provide greater insight into failure and improve accountability. For example, dedicate a pipeline exclusively for building a container image at a time. After that, to run the container instance, you can perform the testing, promoting, and deploying of that image.
6. Stateless Processes
The 12-factor app methodology allows you to run cloud-native applications in the environment as one or more processes. The only restrictions are that they should be stateless and never share data. That enhances scalability and portability across cloud computing infrastructure. Data compilation is done during the build stage. Any other thing that requires persistence forms part of the backing services.
Containers are short-lived and when the container goes away, the data inside the container ceases to exist. The state of containerized workloads must be reduced. This helps to maintain a good user experience while remaining unaffected by application scaling.
7. Port Binding to Export Services
This stage of 12-factor app development involves binding your packaged application to a port. You can use the Kubernetes service object if the workload is exposed internally to the cluster. Otherwise, you need other methods such as node ports, Ingres Controllers, and OpenShift routes.
Packing your application inside containers makes networking and port collisions easier by reducing the workload on hosts. Software-defined networks in Kubernetes platforms take over many operations.
8. Concurrency and Scalability

Scalability is one of the primary features of any cloud-native application. That is usually done by deploying more app copies instead of enlarging them. To achieve this, the 12 factor app methodology uses a simple yet reliable operation.
The developer designs the app to take on different workloads by assigning processes varying tasks. An example is an application where a web process handles HTTP requests and a worker processes a long-running background activity.
The pod-based Kubernetes architecture supports the scaling of application components as per varying demands. With the 12-factor app’s stateless processes element, scalability becomes a consistent function that can help to gain an expected level of concurrency.
9. Disposability: Robust Cloud-native apps
According to the 12-factor app methodology, all processes are disposable. They should have minimal startup time, shutdown gracefully, and be immune to crashes and failures. All these capabilities make scaling easier, enhance faster app development, and make the deployments more robust.
The app should create new instances when it needs to and take them down as necessary. It is this 'disposability' property that makes cloud-native applications more robust. In microservices, processes are disposable. That means, in case any application stops working unexpectedly, users stay almost unaffected and the failures are managed gracefully. You can also use Kubernetes ReplicaSets to uphold the stage of availability for microservices by specifying the max-to-min bounds for the number of replicas.
10. Dev/prod parity: Carrying out Development and Production Similarly
The 12-factor app methodology bridges the gap between cloud-native app development and production. That makes it possible to continuously deploy or roll out new features. Also, developers can write code, deploy, and review the app’s features. This process is usually fast, and completed in minutes or hours.
For organizations that pack workloads into containers, initializing the container image in one environment, it shall run on any infrastructure or environment. But there are chances of environmental drift. Consider standardizing the same distribution of Kubernetes across all environments to eliminate this. It helps create a consistent experience for container platform users.
11. Logs are Event Streams
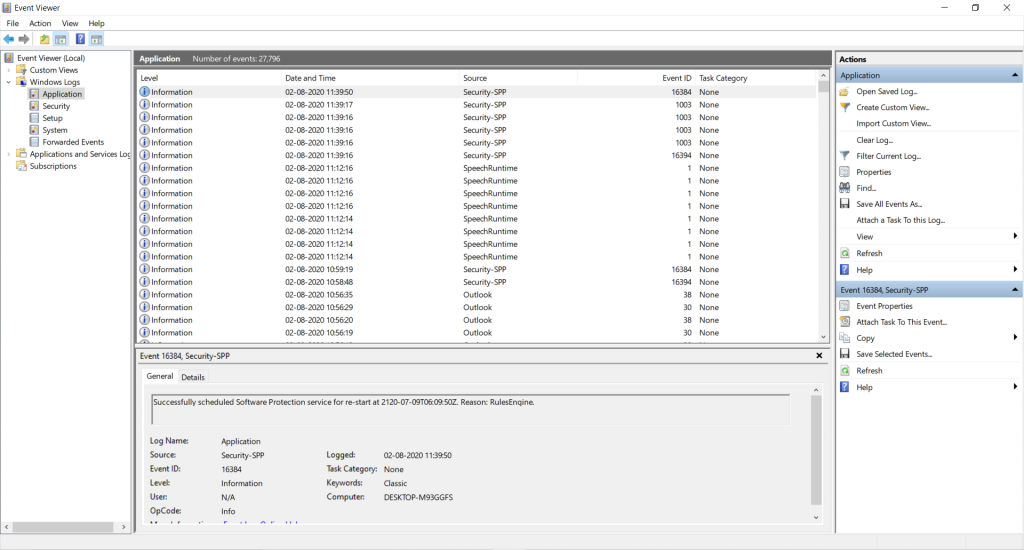
The 12-factor app methodology does not require routing and storage, writing, or managing of the application’s output or log files. Any running process writes its event stream to STDOUT without buffering. A developer views this stream in the foreground of their user interface. This helps to determine how the app behaves and draw conclusions. The event streams also make it easy to troubleshoot or debug an application.
12. Run Admin Processes as One-off Processes
With a 12-factor app methodology, admin and management tasks such as database migrations, scripts, and batch programs run as one-off processes. They are treated as long-running processes. They also utilize the same dependency isolation methods as the app’s usual processes.
It is best practice to isolate the application administrative tasks such as data restore and backup, caching, or migration from the application microservices and carry them out as separate processes. You can use Kubernetes jobs to execute these mundane administrative tasks that are part of the application lifecycle.
What are the Business Benefits of 12 Factor App Methodology
The 12-factor app methodology is the how-to guide for creating cloud-native applications. Many giant tech companies, such as Amazon, Heroku, Microsoft, and others, make use of these 12 principles as they technically help them to enhance business agility by expediting innovation and go-to-market capabilities. With these 12-factor principles, you can design and maintain a robust and modern app architecture required for cloud-based applications.
This methodology is the solution for developers developing the following:
Software-as-a-service solutions
|
Cloud Applications
|
Distributed software solutions
|
Microservices
|
With the 12-factor apps methodology, you can create cloud-native applications that are:
- Suitable for deployment on modern cloud platforms, minimizing the need for servers and server administration
- Enabled for continuous deployment with minimal differences between development and production
- Scalable without significant change or effort
- Capable of using declarative formats for setup automation.
Conclusion
Web applications, platforms, and frameworks using the 12-factor app methodology have generated measurable business outcomes with enhanced productivity in the past few years. This guidebook is suitable for DevOps and cloud app development, which should be a blueprint for developing resilient, scalable, portable, and maintainable applications. Considering these 12-factor app principles with Kubernetes ensures you build a robust solution for your business.
However, this methodology is not the ultimate solution for everyone. Whether or not it works for your business depends on your business model and needs. So, you should not worry if your software development process deviates from the principles of 12-factor app methodology. You are good to go if you understand the reason and the expected outcome.
Do you want to learn more about 12-factor app development principles and its real-time use cases? Contact us today to know how we can help your company.
Book 1-hour free consultation